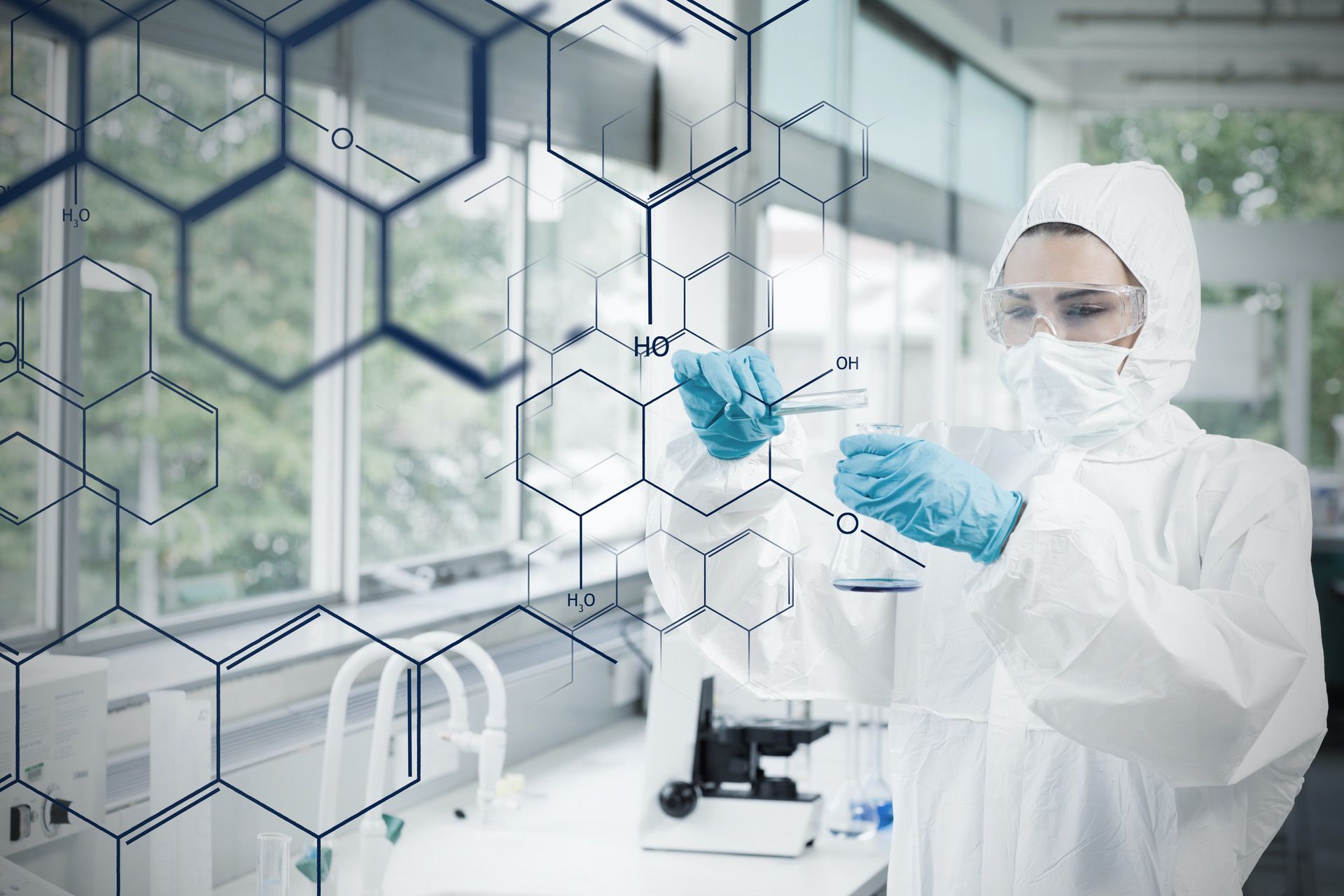
What it is, when it makes sense
Quality problems are still affecting a portion of chemical companies, driving costs and leadtimes up through rework, rejects, and scrap. Six-Sigma and Lean helped identifying the major causes for poor quality – being varying specs of raw materials, inaccurate weighing, deviations from SOPs, inadequate or worn-out equipment, uncalibrated QC-devices, and so on. However, traditional quality improvement methods required long timespans – e.g. for root-cause analysis, Design of Experiments, piloting, etc. – until results materialize and hit the bottom line. New big data and predictive analytics methods have the potential to significantly shorten these leadtimes through faster / better simulation capabilities, algorithm automation and higher computational power.
What you get
Our typical „Quality 4.0 with computational chemistry” engagement delivers five items:
- Baseline quality losses and potentials/priorities, i.e. the identification of the starting point in terms of quality performance and losses, the analysis of existing production processes and workflows, as well as which product lines or areas the project should focus on to capture the biggest benefits first.
- Roll-out plan, i.e. the formalization of actions required to complete the project, the staffing, the costs – including cost-benefit analysis.
- Technology scouting, i.e. the identification of technologies necessary to support the process (e.g., predictive analytics software, retrofit sensors and infrastructure), the selection of vendors & negotiations.
- Technology configuration, i.e.tailoring and configuration so that it fits the specific requirements of the plant.
- Predictive analytics methods defined, tested, and in use, e. the selection, testing, and formalization of the algorithms and workflow that shall drive quality predictions in the future, as well as the training of key personnel / experts to run predictions on their own.
- Quality management system upgrade, e. adaption of the existing management processes (e.g. weekly / monthly quality meetings) and operational processes (e.g. QC testing on raw materials, SOP approval / adaption, batch release, QC testing on WIP and finished goods) to include changes from the adoption of the predictive analytics approach.
How we work
We believe that „the value is at the interfaces“ between business (industry and functional perspective) and technology (software, hardware, workflow perspective), and staff our projects to replicate this variety. Our typical project in this area therefore staffs:
- An expert in chemical manufacturing & quality
- An expert in predictive analytics, big data, and machine learning
- A digital project manager with experience in agile project management methods – often this role is exercised by the manufacturing expert
- An expert from the selected software vendor to help configure the tools
and lasts between 3 and 12 months, depending upon the scope of action.
Our way of working is different from traditional consultancies and from technology vendors, and is characterized by:
- Highly collaborative style, with frequent, spontaneous workshops, trainings, and joint exercise & tests
- Agile project management methods, such as focus on realization („Minimum viable solution“, design thinking principles like desirability, feasibility, viability) and high-pace drumbeat through weekly milestones („sprints“)
- An orientation towards impact („If it does not bring something, let´s drop it“)